Case Study: Amazon's Mastery in Production Problem-Solving
- SefasTech Editorial Team
- Feb 16, 2024
- 2 min read
At the core of Amazon's problem-solving approach is the embrace of automation and data analytics. The company's decision to fully automate certain product categories around 2014 marked a bold step into optimizing its retail business. This move was driven by a willingness to take risks and a strong belief in the power of technology to improve efficiency and customer satisfaction. Their venture into complete automation of certain product lines demonstrated significant improvements in customer experience outcomes, such as in-stock rates, while also reducing costs.
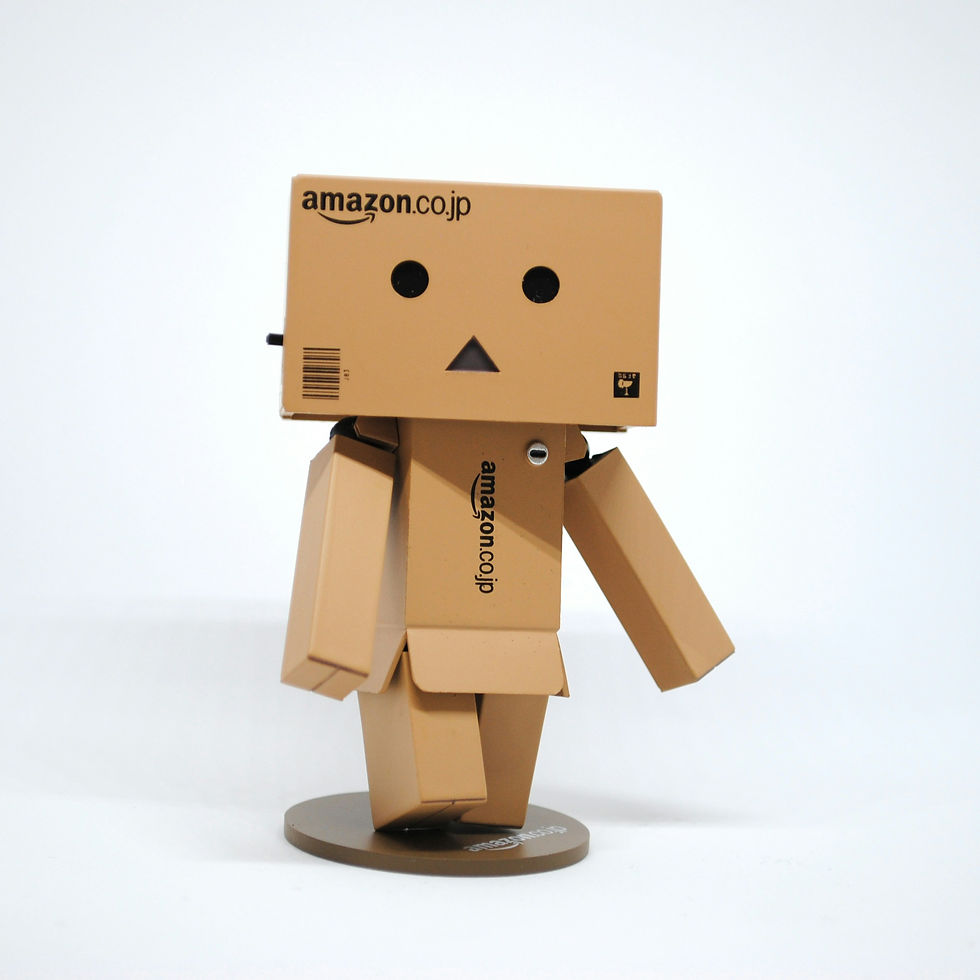
Amazon's Supply Chain Optimization Technologies (SCOT) team played a pivotal role in this transformation. The team focused on developing technologies that would enable seamless connections across different parts of the Amazon supply chain. One of their notable achievements is the development of a unified forecasting model, which incorporates deep learning to accurately forecast shifting seasonal demands and future event-related demand spikes. This model helps Amazon to manage product flows worth hundreds of billions of dollars, making precision in predictions crucial.
The company faced additional logistical challenges with its ambitious customer delivery promises, such as the One-Day Delivery in the US and Prime Now. To address these, Amazon used detailed SCOT simulations, like the Mechanical Sensei, to determine the additional inventory needs, optimal placement, and the impact on shipping costs. The complexity of adding local warehousing and determining which items should be locally available was another challenge that Amazon tackled by redesigning its automated network. This included a multi-echelon system for optimizing inventory levels for varying delivery speeds in an environment where future demand and capacity constraints are uncertain.
Amazon's problem-solving extends to its Fulfillment by Amazon (FBA) system, which empowers sellers with more control over their capacity within Amazon’s fulfillment network. The company has also been innovating in forecasting methods, evolving from generating peak computation-load forecasts to creating per-minute forecasts several months into the future.
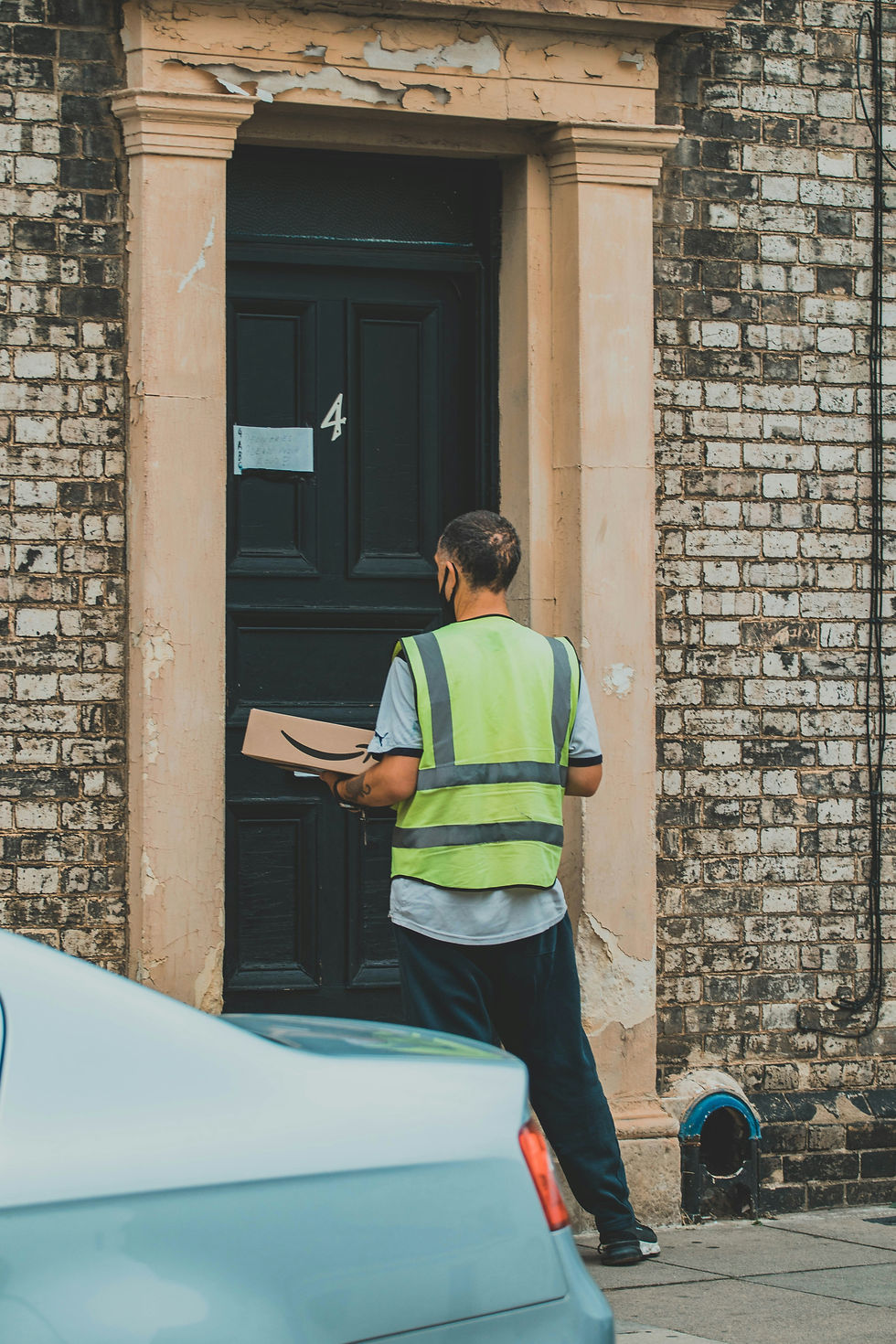
Despite these advancements, Amazon continues to face and address new challenges. For example, balancing the supply and demand of capacity in a network with a significant portion of inventory coming from FBA sellers remains a complex business problem. Amazon's SCOT team is actively working on inventing new approaches to offer predictability to sellers while balancing the network to ensure a wide in-stock selection for customers.
In summary, Amazon's approach to production problem-solving is a blend of embracing automation, harnessing the power of data analytics, and continuously innovating to adapt to new challenges. The company's journey in optimizing its supply chain and fulfillment processes provides invaluable insights into the potential of technology and data-driven strategies to transform business operations.
Comments